Partnerships are increasingly essential in scaling business operations, extending reach, and driving innovation. With the explosion of data, shifting customer expectations, and the complexity of managing multiple collaborators, Artificial Intelligence (AI) has emerged as a vital enabler. Implementing AI in partnership ecosystems enhances collaboration, decision-making, and performance optimization.
Bringing AI into partnership ecosystems integrates AI with data, security, and applications to enhance overall effectiveness and impact, supporting developers in adopting AI technologies within different facets of development.
However, successful implementation requires more than plugging in a tool. It demands a cohesive strategy grounded in business needs, clear objectives, and organizational readiness. This blog provides a comprehensive guide to the strategies organizations should adopt when implementing AI solutions specifically for managing partnerships.
Define the Purpose of AI in Partnerships
Before diving into implementation, it is crucial to define the business goals AI should achieve within the partnership landscape. Organizations should:
- Identify pain points or inefficiencies in existing partnership workflows.
- Clarify what outcomes they expect from using AI (e.g., faster onboarding, smarter partner segmentation, predictive analytics for partner performance).
- Set measurable KPIs to gauge success.
Clearly articulating the problem AI is meant to solve creates alignment and focus.
Understand the Types of AI Capabilities for Partnerships
AI is not a monolithic tool—it includes various technologies that serve different purposes. Businesses need to choose the right type of AI for their partnership challenges. These include:
- Machine Learning (ML): Identifies patterns in partner behavior, forecasts trends, and enables smart segmentation.
- Natural Language Processing (NLP): Analyzes communications, supports chatbots, and mines partner feedback from emails or reports.
- Robotic Process Automation (RPA): Automates repetitive tasks like data entry, contract review, or report generation.
- Predictive Analytics: Provides insights into future partner performance, renewal likelihood, or market fit.
- AI-based Recommendation Engines: Suggest next best actions, partner programs, or marketing campaigns based on historical data.
- Digital Assistant: Improves customer experiences by providing personalized support and efficient service, making it a competitive and cost-efficient option for tasks like math and coding, as seen with models like DeepSeek-R1.
Understanding these capabilities helps determine which technologies best support your strategic goals.
Evaluate AI Readiness Across the Organization
Implementing AI requires more than interest and budget. Organizations must evaluate if they’re structurally and culturally ready. This includes:
- Data Infrastructure Readiness: AI depends on quality data. Ensure your systems can collect, store, and clean partnership-related data effectively, and provide easy access to this data. Easy access to quality data enhances analytics and AI workloads, promoting experimentation with foundational models and ensuring secure and scalable operations within AI services.
- Workforce Preparedness: Your team should understand AI basics. Invest in training or hire professionals with AI experience.
- Cross-Department Collaboration: AI in partnerships touches marketing, sales, legal, and IT. Alignment across teams is critical.
- Change Management: Staff must be prepared to adopt new processes and technologies without resistance.
Without organizational readiness, AI efforts can falter due to lack of support or infrastructure.
Prioritize Use Cases for Maximum Impact
AI implementation should start with focused, high-impact use cases before expanding further. Potential use cases in partnerships include:
- Partner Scoring and Segmentation: AI models can assess and classify partners based on past performance, engagement, or fit.
- Automated Partner Onboarding: NLP-powered systems can answer questions, complete paperwork, and guide new partners through onboarding.
- Contract Analysis: AI can scan partnership contracts for risks, obligations, and inconsistencies.
- Pipeline Prediction: Predict which partner-led deals are most likely to close.
- Marketing Optimization: Suggest personalized campaigns based on partner behavior and preferences.
Select use cases with clear ROI, availability of data, and stakeholder buy-in to demonstrate early wins. Additionally, optimizing and scaling AI workloads is crucial for efficient and resilient management of AI-related processes.
Design an AI Architecture That Supports Scale
AI architecture must be scalable, flexible, and integrative. Consider:
- Modular Design: Build AI capabilities as independent modules that can be upgraded or replaced without overhauling the entire system.
- APIs for Integration: AI must integrate with your CRM, PRM, marketing automation tools, and data warehouses.
- Cloud-Native Platforms: These offer flexibility, computational power, and speed to process large datasets.
- Security and Compliance: Include measures to handle partner data securely and meet legal standards (GDPR, CCPA, etc.).
A strong technical foundation ensures your AI investment grows with your business.
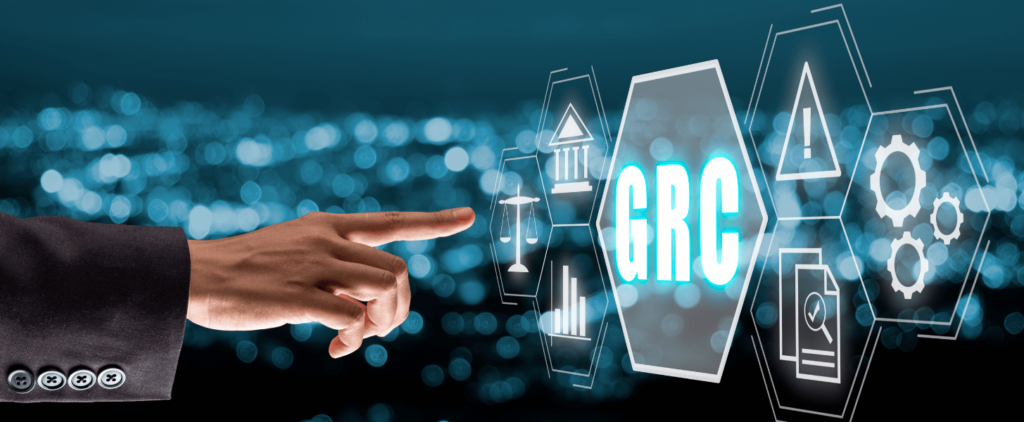
Establish Governance and Ethical Guidelines
AI in partnerships can impact decisions, resources, and partner experiences. Proper governance ensures that AI is used responsibly. Strategies include:
- Create an AI Ethics Committee: Involve leadership, legal, and partner-facing teams.
- Implement Bias Detection: Regularly review AI outputs for bias in partner selection or engagement.
- Transparency Requirements: Make sure AI-generated recommendations or scores are explainable.
- Consent and Privacy Controls: Partners should know how their data is used and have the option to opt out.
Additionally, offering a range of AI-related services, including customized subscriptions and as-a-Service models, ensures clients can access tailored support and resources for effective implementation across various business domains.
Responsible AI usage enhances trust and long-term value.
Foster Continuous Learning and Improvement
AI thrives on feedback loops. As the ecosystem evolves, so should your AI models. Practices include:
- Model Monitoring: Regularly track model accuracy and relevance.
- Fine Tuning: Optimize model performance to meet specific needs, enhancing effectiveness through advanced features.
- A/B Testing: Experiment with new models or algorithms before full deployment.
- Partner Feedback Mechanisms: Use surveys or digital tools to get real-world feedback on AI-driven tools.
- Cross-Functional Review Panels: Include stakeholders from partner management, sales, and marketing to evaluate AI outcomes.
This iterative approach ensures sustained performance and relevance.
Invest in the Right Talent and Partnerships
Successful AI implementation for partnerships requires collaboration among internal teams and external experts. Build or acquire:
- AI Engineers and Data Scientists: To build, train, and optimize models.
- Partnership Strategists: Who understand both human and AI-driven processes.
- Tech Vendors or Consultants: Who specialize in AI implementations.
- Integration Specialists: To ensure smooth connectivity across your tech stack.
Strategic hiring or partnering bridges capability gaps. Staying informed about industry news and collaborating across sectors drives innovation.
Align AI with Broader Partnership and Ecosystem Strategy
AI tools must complement—not replace—strategic alliance efforts. Embed AI within your larger ecosystem roadmap:
- Use AI insights to inform partner recruiting and engagement strategies.
- Let AI suggest co-marketing or co-selling opportunities based on data.
- Connect AI outputs with human alliance managers to ensure balance. Utilize the most valuable data that resides on-premises and at the edge to fuel AI innovation.
AI should support your partnership goals, not drive them in isolation.
Build an AI-Centric Culture Within Partnership Teams
Cultural alignment is key to successful AI adoption. Encourage a data-driven mindset by:
- Hosting AI learning sessions tailored to partnership professionals.
- Celebrating successful AI-led projects.
- Training staff on how to interpret and act on AI insights.
- Building dashboards that make AI findings accessible to non-technical users. AI also helps improve efficiencies by automating routine, everyday tasks.
A culture that embraces experimentation and analytics will extract maximum value from AI.
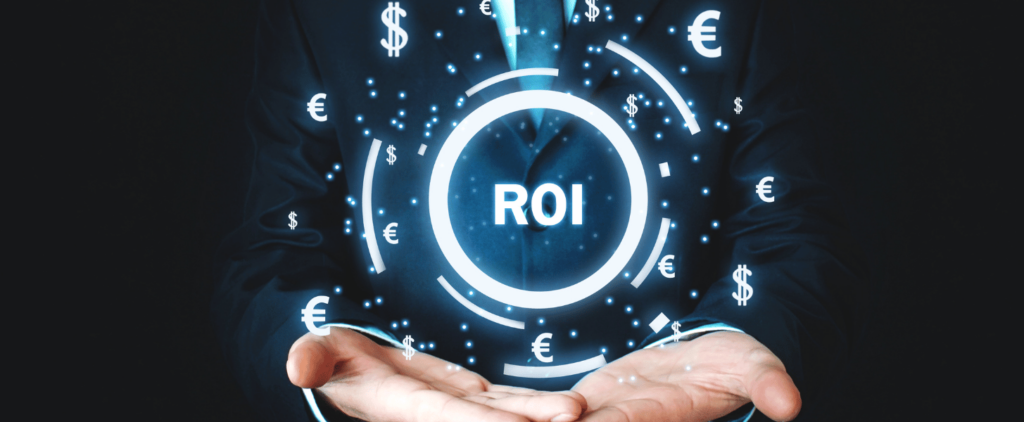
Track and Report ROI of AI in Partnerships
To ensure ongoing investment and stakeholder support, track:
- Partner activation and retention rates.
- Reduction in onboarding time.
- Campaign effectiveness with AI-personalized recommendations.
- Partner satisfaction and experience scores.
- Operational efficiencies and cost savings.
Highlighting real results achieved through AI technology can demonstrate the practical effectiveness of these solutions, showcasing tangible outcomes and successes.
Create simple, visual dashboards to communicate results across departments.
Avoid Common Pitfalls in AI Implementation
AI implementations often falter due to avoidable mistakes. Watch for:
- Overambition: Trying to solve too many problems at once.
- Data Silos: Without integrated data, AI insights will be fragmented.
- Lack of Change Management: Teams unprepared for new workflows may resist adoption.
- Ignoring Partner Experience: Overreliance on automation can alienate partners.
- Specific Audiences: Generating diverse content tailored for specific audiences is crucial. Machine-learning algorithms can analyze patterns and existing data to produce various types of media that cater to the needs and preferences of these targeted groups.
Addressing these proactively ensures smoother adoption and long-term success.
Future Trends in AI for Partnerships
Staying ahead means anticipating what’s next. Emerging trends include:
- AI-Powered Ecosystem Intelligence: Tools that map and analyze entire partner networks.
- Autonomous Partner Engagement Engines: Systems that trigger partner actions based on real-time data.
- Generative AI for Content Co-Creation: Creating joint marketing materials or sales decks instantly.
- Conversational AI with Sentiment Detection: Helping partner managers gauge tone and mood from messages.
- Decentralized AI Models: AI running at the edge, protecting partner data sovereignty.
Staying updated helps future-proof your strategy.
Examples of Metrics to Measure AI Impact in Partnerships
Quantifying success helps secure continued investment. Key metrics include:
- Number of leads generated by AI-identified partners
- Time saved on onboarding or reporting tasks
- Partner tier movement based on AI scoring
- Response time improvements through AI-powered communication tools
- Reduction in manual errors via AI-assisted processes
A data-driven measurement approach justifies further scaling. Additionally, AI models can achieve competitive results at a lower cost, significantly reducing operational expenses compared to traditional models.
Implementing an AI solution in partnerships is not just about adopting new technology—it’s a strategic transformation. It begins with clarity of purpose, alignment across teams, careful selection of tools, and a willingness to experiment and iterate.
By adopting these strategies, organizations can enhance partner experiences, optimize operations, and build more resilient and data-driven ecosystems. AI isn’t replacing partnership professionals; it’s empowering them with the insights and efficiencies needed to scale impact.
The successful marriage of AI with human strategy is the future of alliance and ecosystem growth.
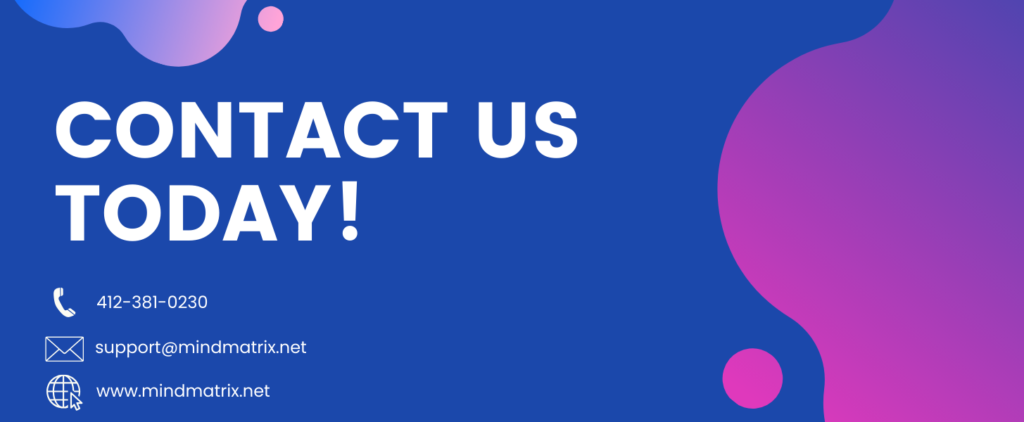